Technologies and platforms for Artificial Intelligence
Technologies and platforms for Artificial Intelligence
The MOOC aims to present the main platforms and technological solutions in the Machine and Deep Learning field.
This MOOC was produced as part of the Edvance project – Digital Education Hub per la Cultura Digitale Avanzata. The project is funded by the European Union – Next Generation EU, Component 1, Investment 3.4 “Didattica e competenze universitarie avanzate".
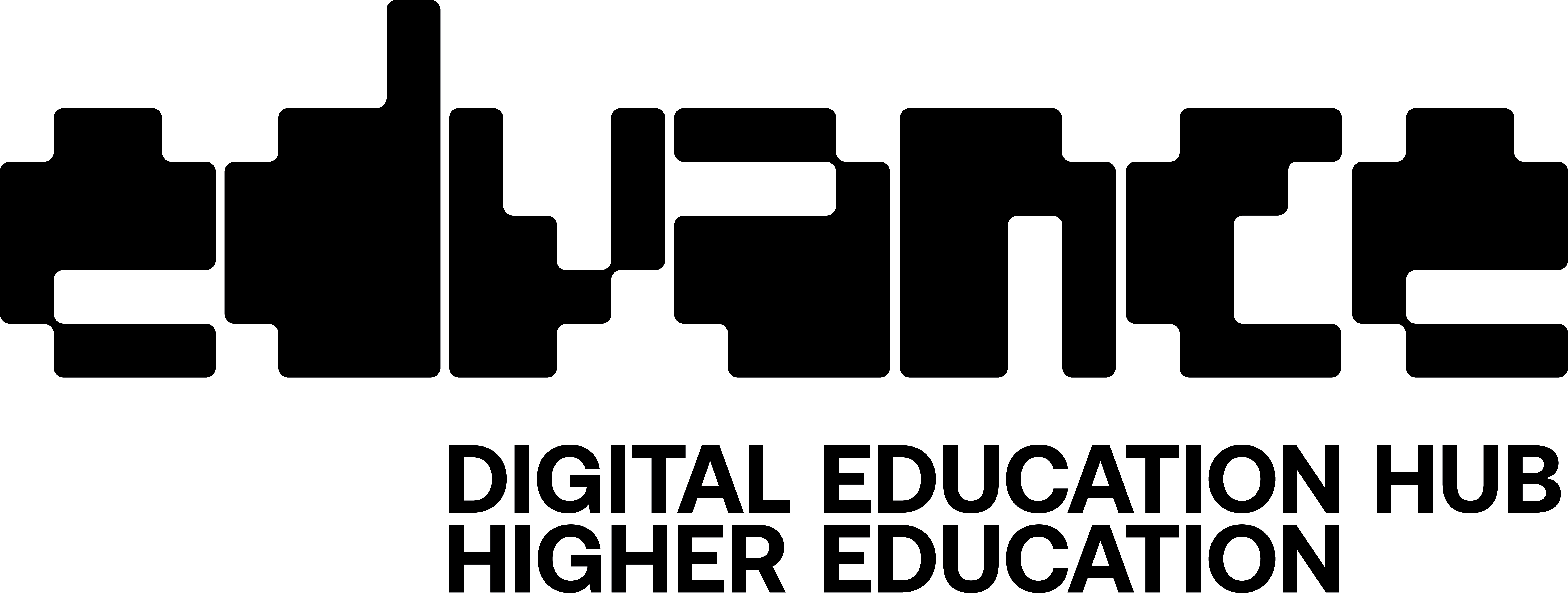
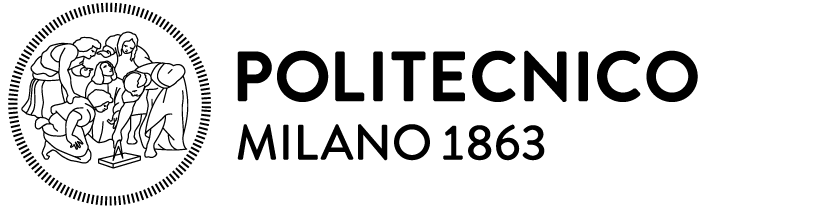
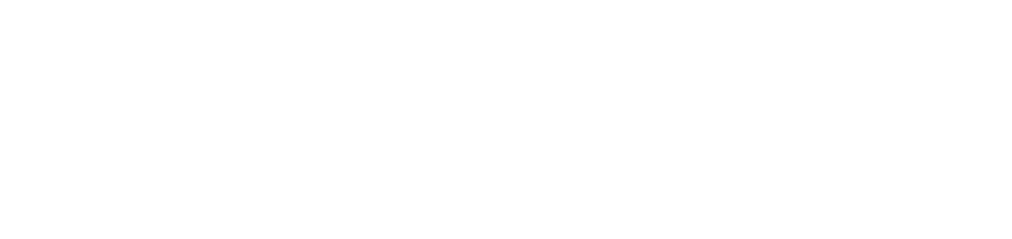
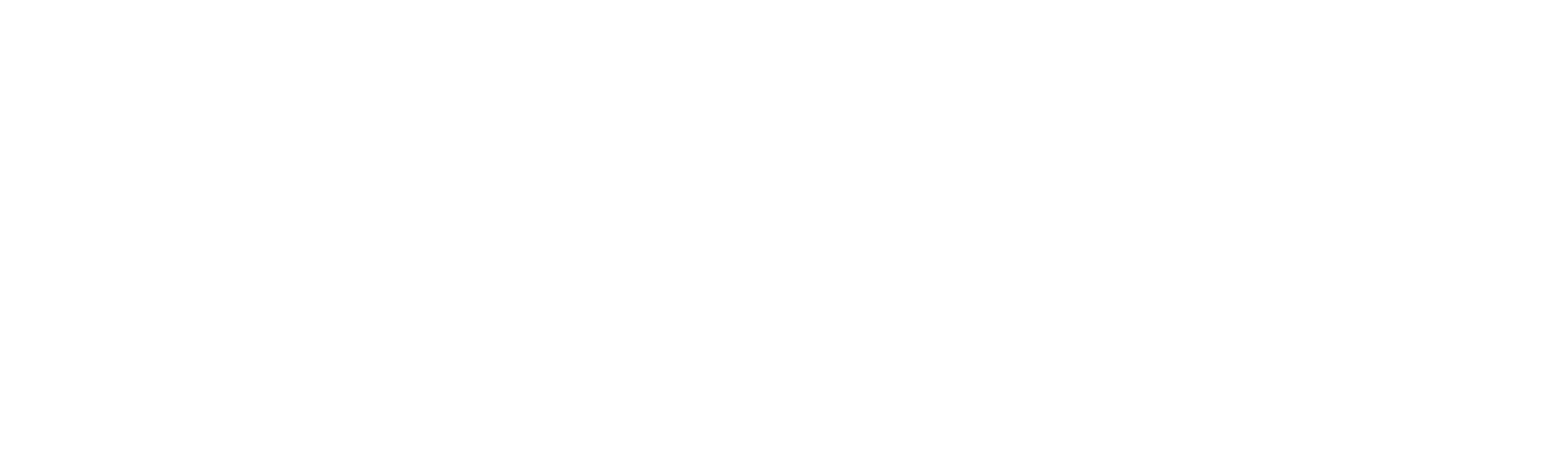
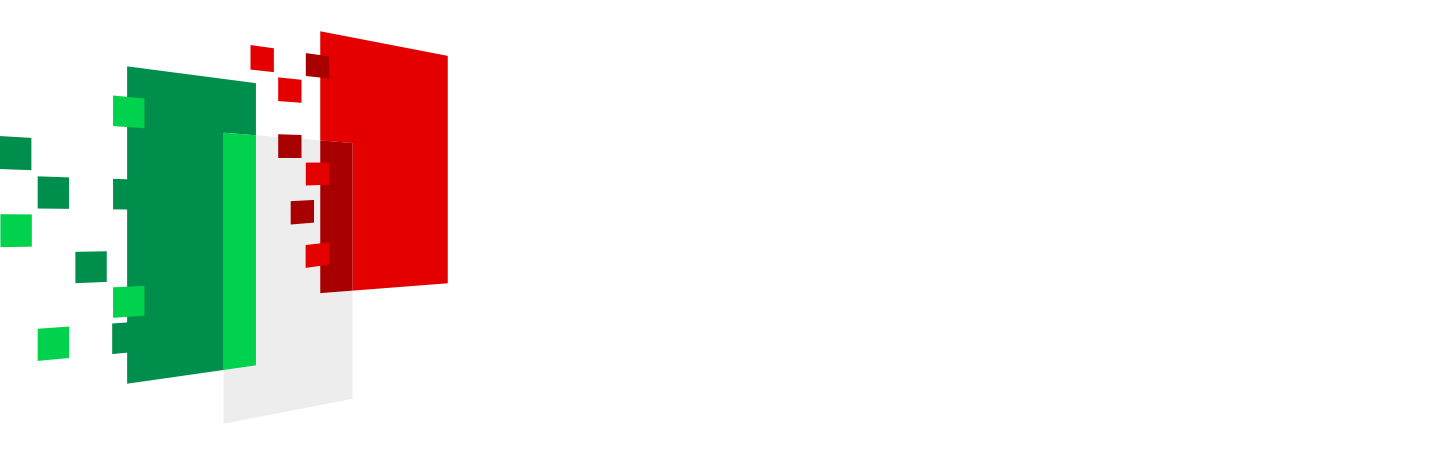
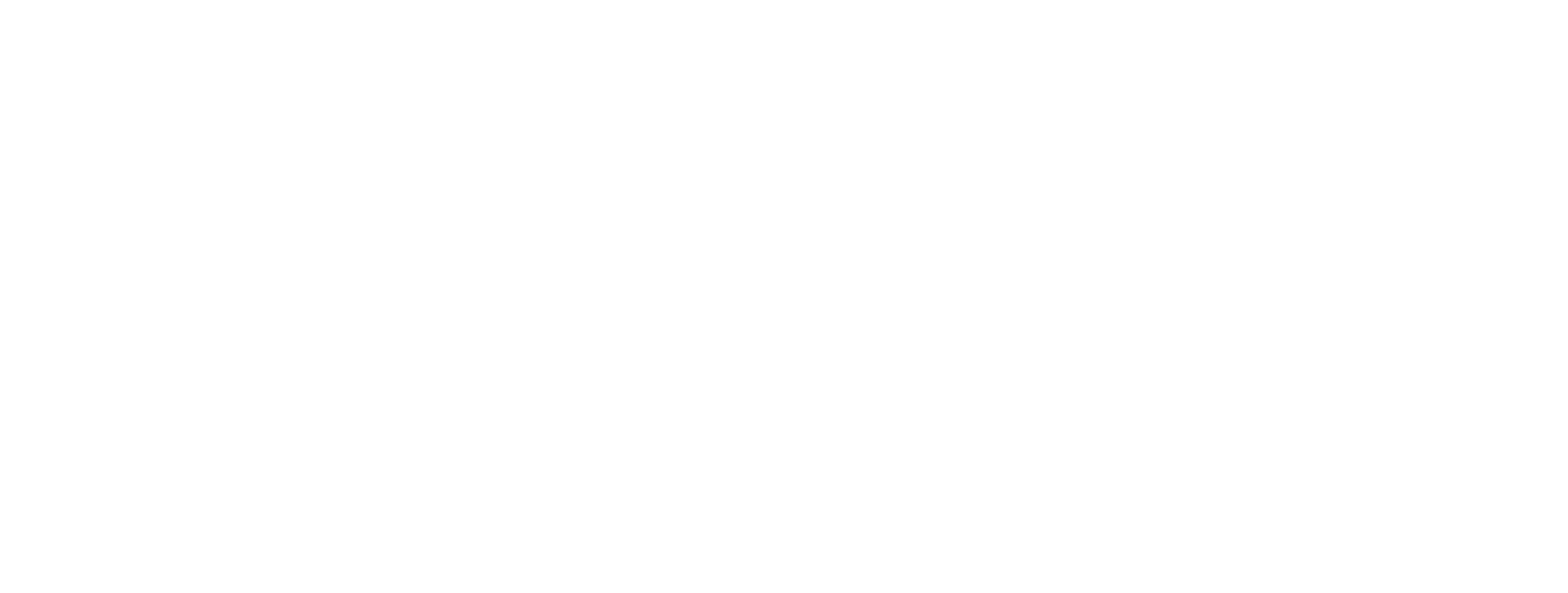
Introduction to Artificial Intelligence Series
This MOOC is one of the MOOCs of the series titled “Introduction to Artificial Intelligence”, aimed at providing technical and non-technical, including historical and political, notions on artificial intelligence. The series investigates why artificial intelligence is nowadays considered the most disruptive enabling technologies up to at least 2050 and gives basic groundings for a preliminary approach to the area. It also deepens ethical issues and national strategies.
See the full seriesCourse description
The MOOC will address the hardware technologies for machine and deep learning (from the units of an Internet-of-Things system to a large-scale data centers) and will explore the families of machine and deep learning platforms (libraries and frameworks) for the design and development of smart applications and systems.
Total workload of the course: 8 h
This MOOC is provided by Politecnico di Milano.
SDG: 4 - Quality Education
Intended Learning Outcomes
By actively participating in this MOOC, you will achieve different intended learning outcomes (ILOs).
Week 1
- Describe the technological scenario of AI (Cloud, Edge, IoT) from an IT perspective.
ESCO: principles of artificial intelligence ESCO: information and communication technologies not elsewhere classifiedESCO: information and communication technologies (icts)
Week 2
- Explain the Cloud-based approaches for AI comprising machine- and deep-learning-as-a-service.
ESCO: cloud technologies ESCO: deep learning - Describe the role of Hardware Accelerators in the grow of AI.
Week 3
- Identify the Machine and Deep Learning techniques and solutions developed for IoT and Edge Computing systems.
ESCO: Internet of Things ESCO: utilise machine learning
Week 4
- Explain the main challenges and opportunities of technologies and platforms for AI.
ESCO: propose ICT solutions to business problems
Prerequisites
The MOOC is aimed in particular at technical staff in charge of developing or adopting artificial intelligence solutions based on Machine and Deep Learning techniques. However, it may be of interest to all those who wish to better understand the platforms and technological solutions in the Machine and Deep Learning field.
Activities
Over and above consulting the content, in the form of videos and other web-based resources, you will have the opportunity to discuss course topics and to share ideas with your peers in the Forum of this MOOC.
Section outline
-
-
Week 1 explains the IT perspective for AI and describes hardware technologies for AI.
-
Week 2 focuses on AI on the Cloud by exploring the typical architecture of Cloud-based AI applications and the role of AI hardware accelerators (i.e., GPU, TPU and FPGA).
-
Week 3 is about Embedded and Edge AI
-
Week 4 explores challenges and opportunities for AI and technologies.
-
-
-
Linkography Page
-
Assessment
You will receive the final course certificate after answering at least 60% (or more) of the final quiz correctly. The maximum score possible for each quiz is given at the beginning of the quiz. You can view your score in the quiz on your last attempt or on the 'Grades' page. Your final grade for the course will be based on the results of your answers to the assessed quizzes you will find at the end of each week (Weekly Quizzes). You have an unlimited number of attempts at each quiz, but you must wait 15 minutes before you can try again.
Certificate
You can achieve a certificate in the form of an Open Badge for this course, if you reach at least 60% of the total score in the final quiz and fill in the final survey.
Once you have completed the required tasks, you will be able to access ‘Get the Open Badge’ and start issuing the badge. Instructions on how to access the badge will be sent to your e-mail address.
The Badge does not confer any academic credit, grade or degree.
Information about fees and access to materials
You can access the course absolutely free of charge and completely online.
Course faculty
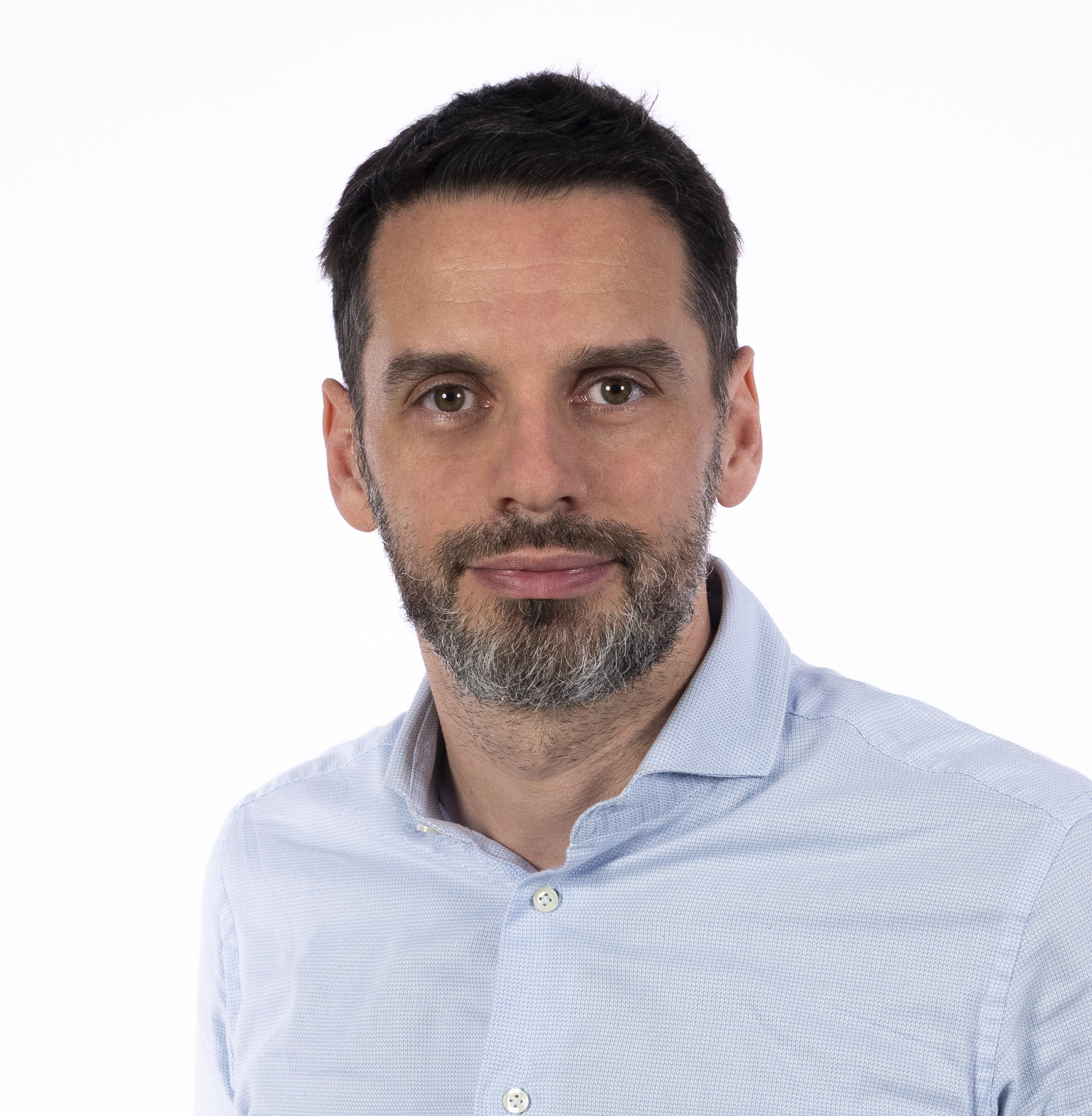
Manuel Roveri
Manuel Roveri received the Dr. Eng. degree in Computer Science Engineering from the Politecnico di Milano (Italy) in June 2003, the MS in Computer Science from the University of Illinois at Chicago (USA) in December 2003 and the Ph.D. degree in Computer Engineering from the Politecnico di Milano (Italy) in May 2007. He has been Visiting Researcher at Imperial College London (UK) in 2011. Currently, he is an Associate Professor at the Department of Electronics and Information of the Politecnico di Milano (Italy).
Current research activity addresses Embedded and Edge Artificial Intelligence, Tiny Machine and Deep Learning, and Learning in nonstationary/evolving environments.
Manuel Roveri is a Senior Member of IEEE and served as Chair and Member in several IEEE Committees. He holds 1 patent and has published about 100 papers in international journals and conference proceedings He is the recipient of the 2018 IEEE Computational Intelligence Magazine “Outstanding Paper Award” and of the 2016 IEEE Computational Intelligence Society “Outstanding Transactions on Neural Networks and Learning Systems Paper Award”.
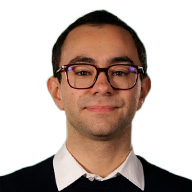
Alessandro Falcetta
Alessandro Falcetta received the Dr. Eng. degree in Computer Science and Engineering in 2018 and the MS in Computer Science and Engineering in 2020, both from Politecnico di Milano, Italy. Currently, he is a PhD candidate in Information Technology at Dipartimento di Elettronica, Informazione e Bioingegneria (DEIB) at Politecnico di Milano, Italy. Current research activity addresses algorithms for Privacy-Preserving Machine Learning and the development of time-series forecasting as-a-service solutions. Alessandro Falcetta is a Member of IEEE.
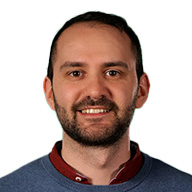
Massimo Pavan
Massimo Pavan received the Dr. Eng. degree in Computer Science and Engineering in 2019 and the MS in Computer Science and Engineering in 2021, both from Politecnico di Milano, Italy. Currently, he is a PhD candidate in Information Technology at Dipartimento di Elettronica, Informazione e Bioingegneria (DEIB) at Politecnico di Milano, Italy. Current research activity addresses algorithms for Tiny Machine Learning and the development of on-device learning solutions for embedded and Internet of things devices. Massimo Pavan is a Member of IEEE.
Contact details
If you have any enquiries about the course or if you need technical assistance please contact pok@polimi.it. For further information, see FAQ page.